At the heart of healthcare, the dialogue between a physician and their patient does more than exchange information—it forms the foundation of diagnosis, management, and the deeply human aspects of empathy and trust. These conversations are where the art of medicine meets the science, requiring a blend of knowledge, intuition, and interpersonal skill to handle the complexities of each patient’s story.
As we continue into unknown technological terrain, it’s not difficult to imagine a future where this interaction is augmented by artificial intelligence, capable of extending the reach of medical expertise to every corner of the globe. In fact, this “future” is becoming our present more and more every day.
Enter Articulate Medical Intelligence Explorer (AMIE): Google’s bold foray into bridging the gap between the capabilities of AI and the age-old practice of medical diagnostics. AMIE isn’t just another AI system; it’s a visionary leap towards enhancing diagnostic dialogues.
By marrying the latest advancements in large language models (LLMs) with the unique demands of medical conversational reasoning, AMIE stands at the forefront of an exciting frontier in healthcare.
But how does one translate the considerable expertise of clinicians into algorithms? How can an AI analyze symptoms and understand the fears, hopes, and needs of patients?
This introduction to AMIE’s world exposes the challenge of approximating human medical proficiency through silicon and code. We are bearing witness to the reimagination of medical consultation.
Today we’ll uncover the inspirations behind AMIE, the intricacies of its development, and evaluate its potential to transform medical diagnostics. Let’s see how AMIE moves beyond simulating conversations into actively learning to think, empathize, and engage in the deeply human art of healing.
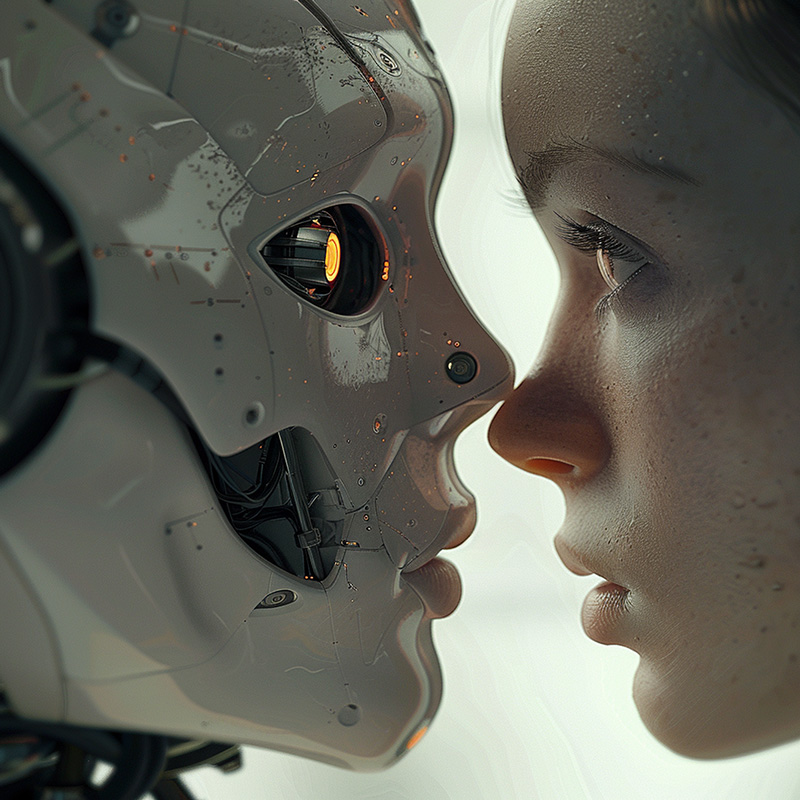
The Challenges of AI Medical Diagnosis
The dance of diagnosis requires a vast store of medical knowledge as well as the ability to apply this information in the context of individual patient histories and symptoms. Clinicians excel in this arena, managing the convolutions of human health with a blend of skill, empathy, and heavy emphasis upon experience that seems, at first glance, beyond the reach of artificial intelligence (1).
An effective clinician doesn’t just ask questions; they create a narrative that combines the patient’s history, current symptoms, and potential concerns into a coherent picture that guides diagnosis and treatment.
Possessing the ability to communicate complex medical information in a clear, compassionate manner is often the only way to build trust and ensure patient compliance with treatment plans.
The challenge of encapsulating this expertise within an AI system appears insurmountable, yet it represents a step forward that cannot be skipped in the quest to enhance healthcare accessibility and effectiveness.
Unique Aspects of Diagnostic Dialogue
For an AI to approach the effectiveness of a human clinician, it must match this nuanced sophistication. At the very least this includes:
- Taking a Complete Clinical History: A combination of data collection and perceiving the implications of the patient’s narrative.
- Intelligent Questioning: Asking the right questions at the right time to uncover pieces of the diagnostic puzzle.
- Empathy and Support: Recognizing and responding to the patient’s emotional state and concerns.
- Clear Communication: Explaining medical concepts and treatment plans in a way that is accessible and reassuring.
The Leap Forward with AMIE
The development of AMIE represents a bold leap toward capturing these aspects. Drawing on the latest advancements in large language models (LLMs), AMIE replicates the depth and breadth of clinical expertise, aiming to hold conversations that diagnose and reassure, educate, and empathize (2).
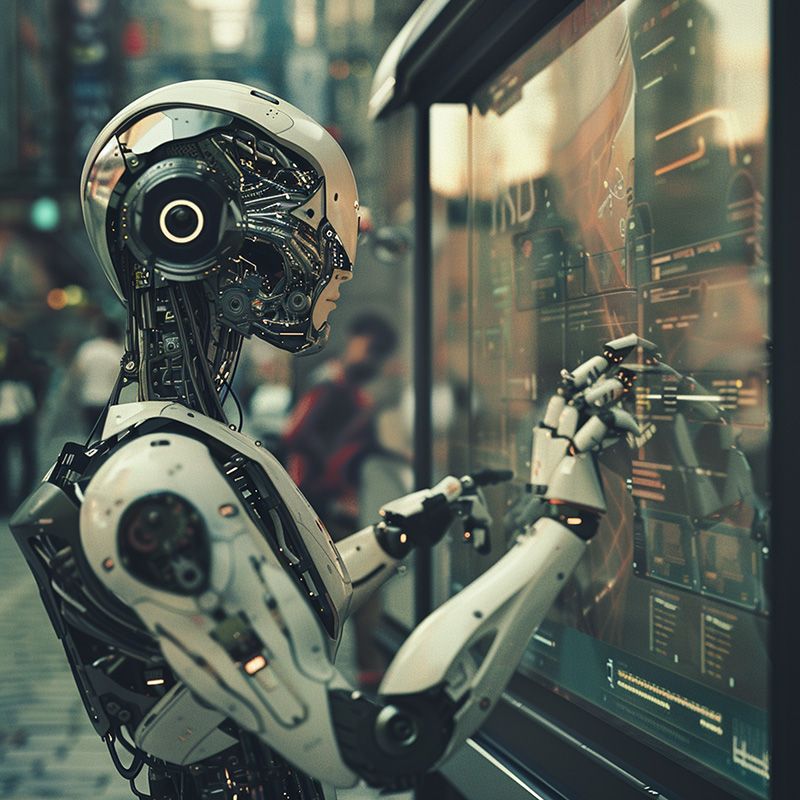
The Genesis of AMIE
AI in Medicine: Inspiration Behind AMIE
AMIE’s inception was driven by the ambitious goal of bridging the gap between the vast potential of artificial intelligence and the empathetic realm of clinical diagnostics. Traditional AI systems have shown promise in various medical applications, from image analysis to patient data management.
However, the complex, conversational interaction inseparable from diagnosis and patient care remained a largely untapped frontier. What made the difference for AMIE was the way in which it was trained.
Beyond Training AI Chatbots: AMIE’s Learning Data
Here’s a breakdown of how AMIE was trained, aiming to maintain a human-like quality in the explanation:
Foundation on Large Language Models (LLMs)
- Base Technology: AMIE is grounded in LLMs, which are advanced AI systems capable of understanding and generating human-like text based on the data they were trained on. This foundation enables AMIE to process and generate language that can mimic human conversational patterns (2).
Multidimensional Training
- Real-World Clinical Consultations: Training involved simulating real-world clinical consultations, considering the perspectives of both clinicians and patients. This approach ensures that AMIE can understand and respond to a wide range of medical inquiries and situations (2).
- Self-Play Simulated Dialogues: A novel training method involving self-play within a simulated diagnostic dialogue environment was used. This involves AMIE interacting with AI patient simulators in a virtual care setting, allowing it to practice and improve its diagnostic reasoning and conversational abilities through automated feedback (2).
- Inference Time Chain-of-Reasoning: To further refine AMIE’s diagnostic accuracy and conversational quality, a chain-of-reasoning strategy was employed during the inference stage. This means AMIE can adjust its responses based on the unfolding conversation, ensuring that each reply builds logically on the previous exchange to arrive at more accurate conclusions (2).
Evaluation and Iteration
- Evaluation Rubric: An evaluation framework assessed AMIE’s performance across various dimensions, such as history-taking, diagnostic accuracy, clinical management, and empathy. This rubric was inspired by tools used in real-world medical education and assessment (2).
- Randomized Double-Blind Crossover Study: AMIE’s effectiveness was tested through text-based consultations with patient actors, comparing its performance to that of board-certified primary care physicians. This study setup mimics an Objective Structured Clinical Examination (OSCE), a standardized method for evaluating clinical skills (2).
Continuous Learning and Scaling
- Self-Play Loops: AMIE underwent two types of self-play loops to continuously improve its abilities. The inner loop focused on refining AMIE’s responses based on feedback from simulated patient interactions, while the outer loop involved integrating these refined dialogues into further training cycles (2).
- Scaling Across Conditions: The self-play simulated learning environment allowed AMIE to expand its knowledge across a wide array of medical conditions, specialties, and patient scenarios, overcoming the limitations of static real-world datasets (2).
Achievements
- Performance: In testing, AMIE demonstrated the ability to conduct diagnostic conversations with comparable or superior effectiveness to real primary care physicians across multiple clinically significant metrics, as judged by both medical specialists and patient actors (2).
In essence, AMIE’s training involved a sophisticated blend of real-world data, simulated interactions, continuous feedback, and strategic reasoning adjustments to enhance its capability in providing accurate, empathetic, and engaging medical consultations.
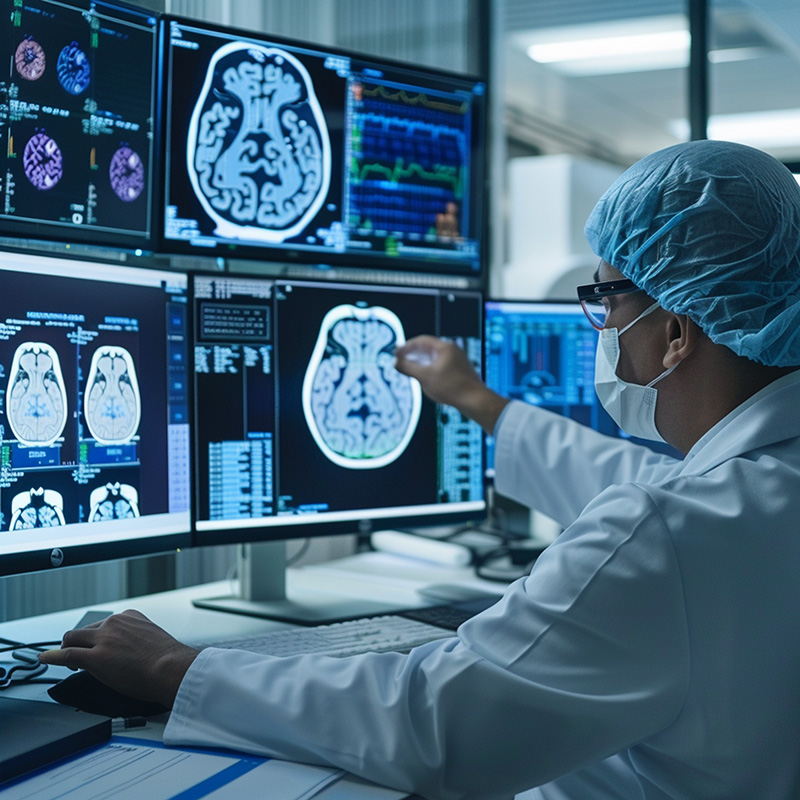
AMIE as a Clinical Aid
A particularly compelling validation of AMIE’s potential comes from its evaluation in the context of complex diagnostic challenges, as reported in a study with the New England Journal of Medicine (NEJM).
Assisted Diagnostic Accuracy in Complex Cases
The NEJM study put AMIE to the test in a rigorous and realistic setting, examining its performance on challenging real-world medical cases. Clinicians, both with and without AMIE’s assistance, were asked to provide differential diagnoses (DDx) for a series of cases sourced from NEJM’s ClinicoPathologic Conferences (CPCs). These cases are known for their complexity, offering a stern test of diagnostic acumen (2).
When assisted by AMIE, clinicians demonstrated a notable improvement in their ability to generate comprehensive and accurate differential diagnoses. AMIE’s performance, evaluated against unassisted clinicians, showcased a top-10 accuracy of 59.1%, compared to 33.6% for clinicians without AMIE (2).
Enhancing Clinician Capabilities
The study’s findings show that AMIE acts as a catalyst for improved diagnostic outcomes. By leveraging this AI model, clinicians can tap into a vast repository of medical knowledge and reasoning, complementing their expertise and experience.
This synergistic relationship between AI and human intelligence opens new avenues for tackling diagnostic challenges, particularly in cases where the complexity might overwhelm the limits of individual experience, in resource-constrained settings, or in situations where rapid decision-making is a matter of life or death.
Toward a Collaborative Future
As we stand on the brink of this new era in healthcare, integrating AI into clinical practice is just beginning. The successes of AMIE, as demonstrated in the NEJM study, offer a glimpse into a future where diagnostic accuracy elevates the overall quality of care, making personalized, high-level medical consultation more accessible to patients around the world.
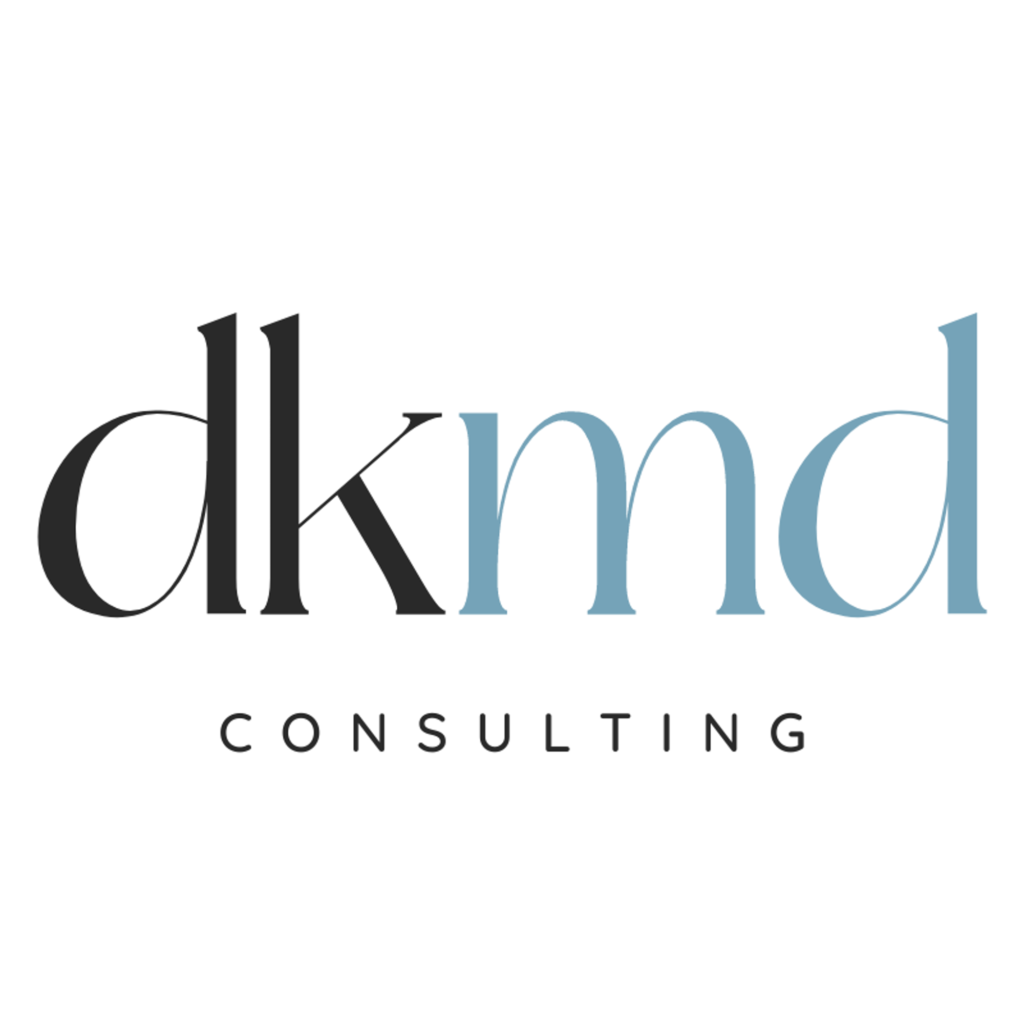
Bringing Clarity and Credibility to Your Medical Content
Artificial intelligence is actively transforming healthcare and the way we communicate about medicine is evolving. Tools like AMIE usher in a new era of collaborative diagnostic excellence, where AI and clinicians work hand in hand to improve patient outcomes.
At DKMD Consulting, we believe the same principle applies to medical writing. Our team of experienced medical writers works closely with healthcare professionals to ensure that every piece of content we produce is scientifically sound, ethically grounded, and genuinely helpful to readers.
We don’t just write about medicine—we help you distill the complexities of medical knowledge into clear, engaging, and trustworthy content. Whether you’re developing patient education materials, publications, blogs, infographics, or white papers, we’re here to help you communicate with authority and integrity.
Partner with DKMD Consulting and let us help you bring the same level of rigor, expertise, and commitment to your medical content that AI-assisted clinicians bring to patient care. Together, we can make a real difference in how people understand and engage with health information.
Ready to elevate your medical content? Contact DKMD Consulting today to learn more about our services and how we can support your mission.
References
- Brush, J. E., Jr, Sherbino, J., & Norman, G. R. (2022). Diagnostic reasoning in cardiovascular medicine. BMJ (Clinical research ed.), 376, e064389. https://doi.org/10.1136/bmj-2021-064389
- Karthikesalingam, A., & Natarajan, V. (2024, January 12). AMIE: A research AI system for diagnostic medical reasoning and conversations. Google Research.