Increasingly, AI is everywhere, from our social media posts to the workplace to virtually every field of medicine. But as AI assimilates into different fields, it comes with a cost.
The carbon footprint of AI is huge and getting huger. That footprint encompasses the considerable amount of hardware needed to facilitate AI and AI training and the energy used in individual AI processes.
As AI and machine learning expand and become increasingly a part of life, their exponential growth could mean exponential harm since global warming is the number one threat to human life itself (1).
On the other hand, AI and machine learning have the potential to save energy, time, and resources in radiology. These advances can offset the energy used and the carbon footprint generated by AI (2).
It’s a classic conundrum. AI can potentially increase efficiency in radiology, therefore using fewer resources. But AI takes up plenty of resources itself. So, is AI in radiology a net plus for the planet, a net minus, or a wash? An article in Radiology describes the issue as a double-edged sword, which is quite accurate. In radiology, much like other areas, AI giveth and taketh away (2).
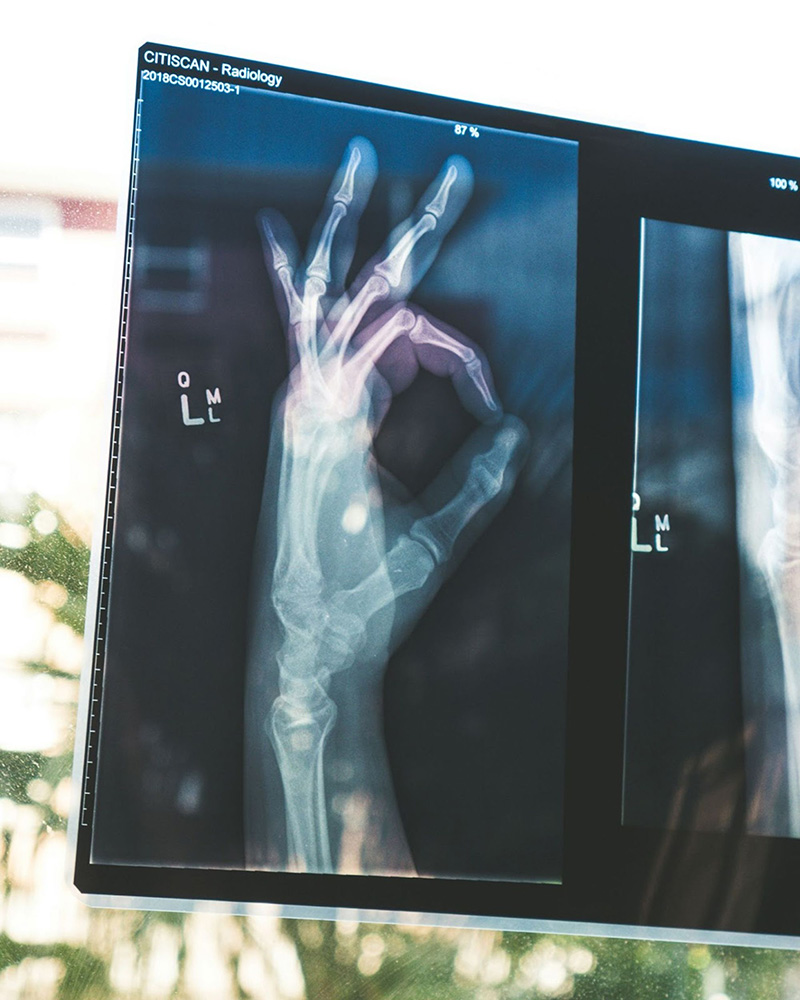
Advanced Radiology: How AI Benefits Radiology Tech
As in the rest of society, AI is increasingly used in radiology, where it naturally enhances existing practices and processes. AI’s familiar ability to manipulate and generate images based on simple commands makes it conducive to any visual field.
There is already a plethora of AI and machine learning technology in radiology, both in research and in the field. In addition to imaging, AI has the potential to influence virtually every aspect of radiology, including diagnosis and research. In areas such as image enhancement and analysis, AI fits radiology like a tailored glove (2).
As Daniel Truhn, et al write in European Radiology, the future potential of AI in radiology is also bright and promising. In particular, radiology may benefit from the creation of foundation models, which are “large multimodal transformer neural networks that can manipulate text, images, and other data types.”
These networks should transform radiology even further. AI is changing everything, but radiology may be one of the most beneficial recipients of that change (2).
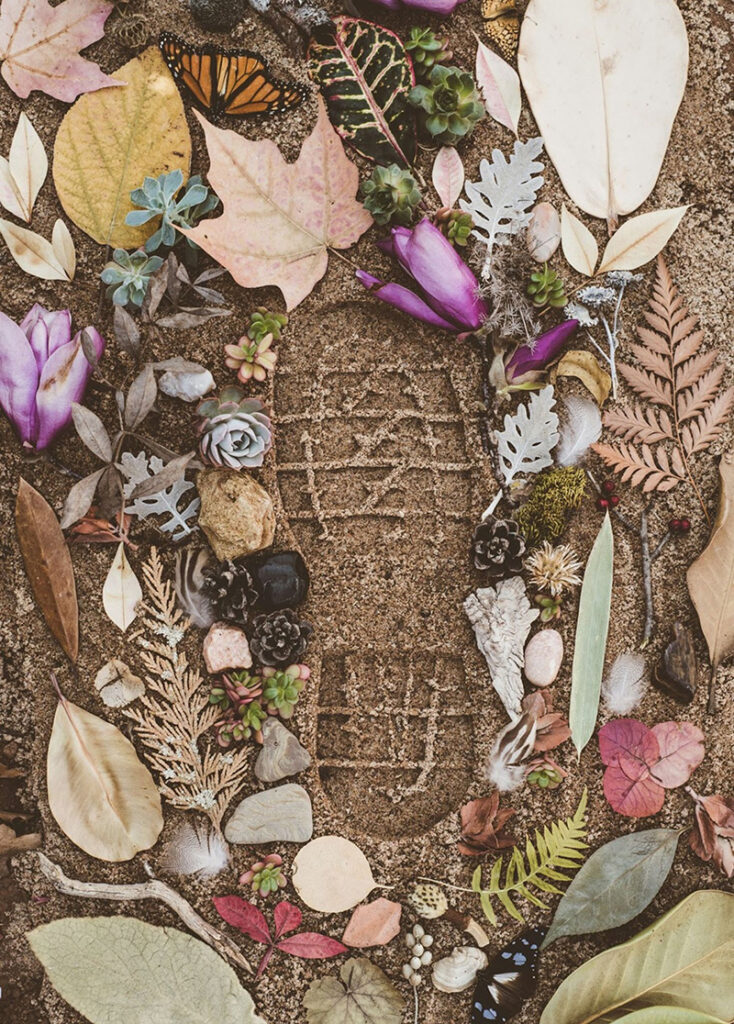
Environmental Impact of AI in Radiology: Understanding Carbon Footprint
Given the resources needed to develop, produce, and support AI, it’s worth looking at the carbon footprint sharply. Both text and image-based AI consume resources, but the study “Power Hungry Processing: Watts Driving the Cost of AI Deployment?” found something startling: generating one image using AI takes the same energy as fully charging a smartphone.
Text-based AI is far more efficient, as the research indicates:
“Text-based tasks are, all things considered, more energy-efficient than image-based tasks, with image classification requiring less energy (median of 0.0068 kWh for 1,000 inferences) than image generation (1.35 kWh) and, conversely, text generation (0.042 KwH) requiring more than text classification (0.0023 kWh). For comparison, charging the average smartphone requires 0.012 kWh of energy, which means that the most efficient text generation model uses as much energy as 16% of a full smartphone charge for 1,000 inferences, whereas the least efficient image generation model uses as much energy as 950 smartphone charges (11.49 kWh), or nearly 1 charge per image generation, although there is also a large variation between image generation models, depending on the size of image that they generate (3).”
In other words, images are far costlier, energy-wise, than text when it comes to AI. Though some use of AI in radiology, such as foundation models, may involve the interaction of image and text, there’s no getting away from imaging as a foundational element of radiology. That’s bad news for AI’s resulting carbon footprint.
Enhancing Radiology Efficiency through Artificial Intelligence
On the other hand, while AI may consume a substantial amount of resources on the back end and in processing, AI also has the potential to reduce the amount of resources needed in radiology through greater efficiency (1).
The environmental impact of medical imaging may actually decrease thanks to AI, if and when the imaging process is streamlined. For example, the less time devoted to imaging a particular scan of a brain, the less resources consumed. This efficiency greatly mitigates the carbon footprint of AI itself—at least potentially so (1).
As Doo FX, et al write in “Environmental Sustainability and AI in Radiology: A Double-Edged Sword:
“AI also has the potential to improve environmental sustainability in medical imaging. For example, use of AI can shorten MRI scan times with accelerated acquisition times, improve the scheduling efficiency of scanners, and optimize the use of decision-support tools to reduce low-value imaging.”
Those are not insignificant advances. Accelerated acquisition time means a faster look at the images doctors need to see. Better scheduling efficiency of scanners could yield significant time and resource savings. And optimization of decision-support tools could lead to the best result of all: better decisions for patients. All of these advances would create better patient and environmental outcomes (2).
A Double-edged Sword, Indeed
Like so many issues, the relationship between AI and radiology and the resultant environmental impact has no simple answer. One could say it’s good and bad for the environment, but that would be facile if technically true.
The work that remains to be done is to study the exact carbon impact of AI and the reduced carbon impact of AI-enhanced radiology, to see how to make the result more conducive to life on earth. In radiology, it remains to be seen how the balance between the good and the bad of AI’s carbon footprint plays out.
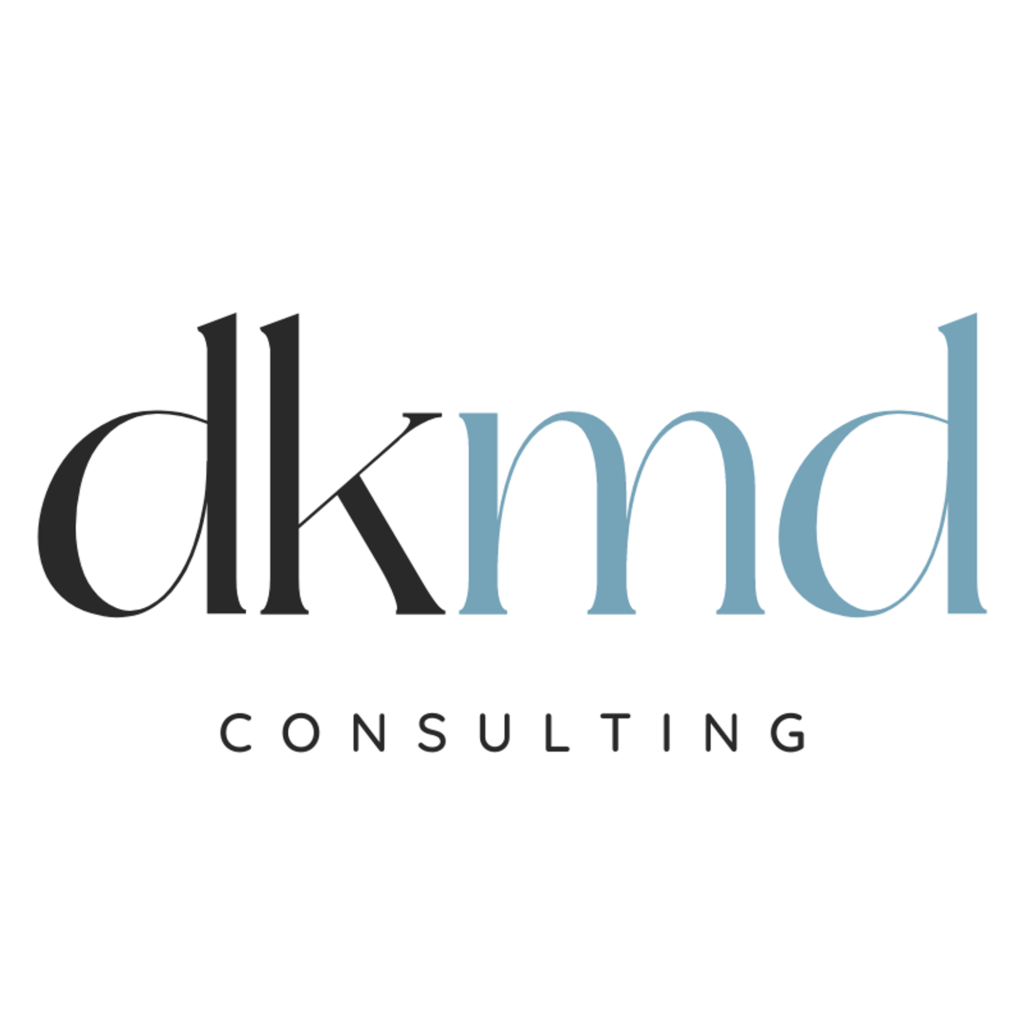
Elevate Your Medical Communications with DKMD Consulting
At DKMD Consulting, we specialize in transforming complex medical innovations, like the recent strides in AI in radiology, into clear, impactful communications that resonate with clinicians and patients alike.
Understanding the intricacies of AI’s impact on radiology can be challenging. Our team excels at distilling this complex information into digestible, engaging content that informs and inspires action.
Whether you aim to educate your clientele about AI’s dual role in enhancing efficiency and its environmental cost or wish to highlight sustainable advances in medical imaging, DKMD Consulting crafts the narrative that aligns with your goals.
Partner with us to enhance how your business communicates about health and science:
- Expert Medical Writing: Benefit from our expertise in medical copywriting to ensure your communications are scientifically accurate and compelling.
- Content Strategy Development: Let us help you build a content strategy that effectively communicates the value of AI in radiology to your audience.
- Educational Materials and Resources: Engage your clients with beautifully crafted educational materials that simplify complex concepts and promote sustainable practices in medical imaging.
Step forward with DKMD Consulting and ensure your communications reflect the sophistication and potential of modern medical science. Contact us today to learn how we can help you articulate the future of healthcare.
References
1) Luccioni, A.S., Jernite, Y., & Strubell, E. (2023). Power Hungry Processing: Watts Driving the Cost of AI Deployment? ArXiv, abs/2311.16863.
2) Doo, F. X., Vosshenrich, J., Cook, T. S., Moy, L., Almeida, E. P. R. P., Woolen, S. A., Gichoya, J. W., Heye, T., & Hanneman, K. (2024). Environmental Sustainability and AI in Radiology: A Double-Edged Sword. Radiology, 310(2), e232030.
3) Truhn, D., Müller-Franzes, G. & Kather, J.N.
(2024). The ecological footprint of medical AI. European Radiology. 34, 1176–1178.